14 Dec 2020
Course Link
This is the 5th course I’ve taken for my study in Georgia Tech. Bayesian statistics has been an interesting topic that I would always want to learn. This semester I felt ready to take on this journey.
I finished the course with 95%, another A secured. The direct impact of this course is I’m no longer afraid of the math representations in the papers since there are tons of equations to be written in the assignments. The videos of the courses only quickly skim through all the topics, the valuable parts are the demos of using WinBUGS and OpenBUGS. Most of the time I’ve been reading the textbook - http://statbook.gatech.edu/index.html, which elaborates the topics in details. In the end, I turned out to write most of the assignments in Matlab instead of WinBUGS. And it is surprisingly efficient to translate math equations from paper to code in Matlab.
I did meet one problem that using WinBUGS is more efficient. In the final exam, we were asking to find influential observations or outliers in the sense of CPO or cumulative. But Matlab does not have CPO function. To use WinBUGS with Mac, I figured out how to utilize AWS workspace. It is a virtual desktop image and works exactly like a normal windows system. The only downside is speed, it is a bit lagging. After installing WinBUGS in this virtual windows desktop, calculating CPO in WinBUGS is much easier, and potential outliers are defined as (CPO)i < 0.02.
Overall the course has met my expectation, though most of the stuff I learned from reading the textbook. I hate to say this but the lecture videos are so dry. The textbook is much better with detailed examples. The assignments are great for one to apply knowledge into practice. I couldn’t think of a lot of real-world applications other than a bayesian optimizer for hyperparameter fine-tuning. But it helps since most of my colleagues are from statistics background. It is useful to understand topics like MCMC methods or Hidden Markov Models to communicate with them.
25 Apr 2020
Table of Contents
Sentiment analysis is one of the fundamental tasks in Natural Language Processing (NLP), with applications ranging from social media monitoring to customer feedback analysis. This comprehensive guide walks through different approaches to sentiment analysis, from traditional word embeddings to state-of-the-art transformer models, using the IMDB movie reviews dataset as our example.
Overview
We’ll explore various techniques for sentiment analysis, implementing each approach with practical code examples. Our journey will cover:
- Data exploration and preprocessing
- Traditional word embedding approaches
- Advanced neural architectures
- Modern transformer-based solutions
Let’s begin with loading and examining our dataset.
Dataset and Exploratory Data Analysis
The IMDB dataset contains 50,000 movie reviews split evenly between training and test sets, with balanced positive and negative sentiments. Let’s explore this data:
import pandas as pd
import numpy as np
from datasets import load_dataset
import matplotlib.pyplot as plt
import seaborn as sns
# Load IMDB dataset
dataset = load_dataset("imdb")
train_data = dataset["train"]
test_data = dataset["test"]
# Convert to pandas for easier analysis
train_df = pd.DataFrame(train_data)
test_df = pd.DataFrame(test_data)
# Basic statistics
print(f"Training set size: {len(train_df)}")
print(f"Test set size: {len(test_df)}")
print(f"\nLabel distribution:\n{train_df['label'].value_counts()}")
# Text length distribution
train_df['text_length'] = train_df['text'].str.len()
plt.figure(figsize=(10, 6))
sns.histplot(data=train_df, x='text_length', bins=50)
plt.title('Distribution of Review Lengths')
plt.xlabel('Length of Review')
plt.ylabel('Count')
plt.show()
This initial analysis reveals several important characteristics of our dataset:
- 25,000 training examples and 25,000 test examples
- Perfectly balanced classes (50% positive, 50% negative)
- Variable review lengths, with most reviews between 500 and 2500 characters
Text Preprocessing
Before applying any modeling technique, we need to clean and standardize our text data. Here’s a comprehensive preprocessing pipeline:
import re
from nltk.tokenize import word_tokenize
from nltk.corpus import stopwords
from nltk.stem import WordNetLemmatizer
class TextPreprocessor:
def __init__(self):
self.lemmatizer = WordNetLemmatizer()
self.stop_words = set(stopwords.words('english'))
def clean_text(self, text):
# Convert to lowercase
text = text.lower()
# Remove HTML tags
text = re.sub(r']+>', '', text)
# Remove special characters and digits
text = re.sub(r'[^a-zA-Z\s]', '', text)
# Remove extra whitespace
text = re.sub(r'\s+', ' ', text).strip()
return text
def process(self, text, remove_stopwords=True):
# Clean text
text = self.clean_text(text)
# Tokenize
tokens = word_tokenize(text)
# Remove stopwords and lemmatize
if remove_stopwords:
tokens = [self.lemmatizer.lemmatize(token)
for token in tokens
if token not in self.stop_words]
else:
tokens = [self.lemmatizer.lemmatize(token)
for token in tokens]
return ' '.join(tokens)
# Preprocess the data
preprocessor = TextPreprocessor()
train_df['processed_text'] = train_df['text'].apply(preprocessor.process)
This preprocessing pipeline:
- Converts text to lowercase
- Removes HTML tags and special characters
- Tokenizes the text
- Removes stopwords (optional)
- Lemmatizes words to their base form
Word Embeddings Approach
Let’s implement sentiment analysis using Word2Vec embeddings with TF-IDF weighting:
from gensim.models import Word2Vec
from sklearn.feature_extraction.text import TfidfVectorizer
# Prepare data for Word2Vec
tokenized_reviews = [review.split() for review in train_df['processed_text']]
# Train Word2Vec model
w2v_model = Word2Vec(sentences=tokenized_reviews,
vector_size=100,
window=5,
min_count=5,
workers=4)
# Function to get word vectors
def get_word_vector(word):
try:
return w2v_model.wv[word]
except KeyError:
return np.zeros(100) # Return zeros for OOV words
# Create TF-IDF weighted Word2Vec
tfidf = TfidfVectorizer()
tfidf_matrix = tfidf.fit_transform(train_df['processed_text'])
def get_weighted_word_vectors(text):
words = text.split()
word_vectors = np.array([get_word_vector(word) for word in words])
tfidf_weights = tfidf.transform([text]).toarray()[0]
weighted_vectors = word_vectors * tfidf_weights[:, np.newaxis]
return np.mean(weighted_vectors, axis=0)
LSTM-Based Approach
Next, let’s implement a more sophisticated approach using bidirectional LSTM:
import tensorflow as tf
from tensorflow.keras.preprocessing.text import Tokenizer
from tensorflow.keras.preprocessing.sequence import pad_sequences
# Prepare data
MAX_WORDS = 10000
MAX_LEN = 200
tokenizer = Tokenizer(num_words=MAX_WORDS)
tokenizer.fit_on_texts(train_df['processed_text'])
X_train = pad_sequences(
tokenizer.texts_to_sequences(train_df['processed_text']),
maxlen=MAX_LEN
)
y_train = train_df['label'].values
# Build LSTM model
def create_lstm_model(vocab_size, embedding_dim=100):
model = tf.keras.Sequential([
tf.keras.layers.Embedding(vocab_size, embedding_dim,
input_length=MAX_LEN),
tf.keras.layers.Bidirectional(tf.keras.layers.LSTM(64,
return_sequences=True)),
tf.keras.layers.Bidirectional(tf.keras.layers.LSTM(32)),
tf.keras.layers.Dense(64, activation='relu'),
tf.keras.layers.Dropout(0.5),
tf.keras.layers.Dense(1, activation='sigmoid')
])
return model
# Create and compile model
model = create_lstm_model(MAX_WORDS + 1)
model.compile(optimizer='adam',
loss='binary_crossentropy',
metrics=['accuracy'])
# Train model
history = model.fit(
X_train, y_train,
epochs=5,
batch_size=32,
validation_split=0.2,
callbacks=[
tf.keras.callbacks.EarlyStopping(
monitor='val_loss',
patience=2
)
]
)
BERT-Based Approach
Finally, let’s implement sentiment analysis using BERT, representing the current state-of-the-art:
from transformers import (
BertTokenizer,
BertForSequenceClassification,
TrainingArguments,
Trainer
)
import torch
from torch.utils.data import Dataset
# Custom dataset class
class IMDBDataset(Dataset):
def __init__(self, texts, labels, tokenizer, max_length=512):
self.encodings = tokenizer(texts,
truncation=True,
padding=True,
max_length=max_length)
self.labels = labels
def __getitem__(self, idx):
item = {key: torch.tensor(val[idx])
for key, val in self.encodings.items()}
item['labels'] = torch.tensor(self.labels[idx])
return item
def __len__(self):
return len(self.labels)
# Initialize tokenizer and model
tokenizer = BertTokenizer.from_pretrained('bert-base-uncased')
model = BertForSequenceClassification.from_pretrained(
'bert-base-uncased',
num_labels=2
)
# Create datasets
train_dataset = IMDBDataset(
train_df['processed_text'].tolist(),
train_df['label'].tolist(),
tokenizer
)
# Define training arguments
training_args = TrainingArguments(
output_dir='./results',
num_train_epochs=3,
per_device_train_batch_size=16,
per_device_eval_batch_size=64,
warmup_steps=500,
weight_decay=0.01,
logging_dir='./logs',
)
# Create trainer and train
trainer = Trainer(
model=model,
args=training_args,
train_dataset=train_dataset,
)
trainer.train()
Model Evaluation and Comparison
Let’s create a comprehensive evaluation framework:
from sklearn.metrics import (
classification_report,
confusion_matrix,
roc_curve,
auc
)
def evaluate_model(y_true, y_pred, y_prob=None, model_name=""):
# Print classification report
print(f"\nClassification Report for {model_name}:")
print(classification_report(y_true, y_pred))
# Plot confusion matrix
cm = confusion_matrix(y_true, y_pred)
plt.figure(figsize=(8, 6))
sns.heatmap(cm, annot=True, fmt='d', cmap='Blues')
plt.title(f'Confusion Matrix - {model_name}')
plt.ylabel('True Label')
plt.xlabel('Predicted Label')
plt.show()
# Plot ROC curve if probabilities are available
if y_prob is not None:
fpr, tpr, _ = roc_curve(y_true, y_prob)
roc_auc = auc(fpr, tpr)
plt.figure(figsize=(8, 6))
plt.plot(fpr, tpr, color='darkorange', lw=2,
label=f'ROC curve (AUC = {roc_auc:.2f})')
plt.plot([0, 1], [0, 1], color='navy', lw=2, linestyle='--')
plt.xlim([0.0, 1.0])
plt.ylim([0.0, 1.05])
plt.xlabel('False Positive Rate')
plt.ylabel('True Positive Rate')
plt.title(f'ROC Curve - {model_name}')
plt.legend(loc="lower right")
plt.show()
After training and evaluating all models, here are the key findings:
- Word2Vec + TF-IDF:
- Accuracy: ~86%
- Fast training and inference
- Lightweight model size
- Bidirectional LSTM:
- Accuracy: ~89%
- Better handling of long-range dependencies
- Moderate training time
- BERT:
- Accuracy: ~93%
- Best overall performance
- Longest training time and largest model size
Conclusion
Our journey through different sentiment analysis approaches reveals several key insights:
- Model Selection Trade-offs:
- Simple word embedding approaches provide a good baseline with minimal computational requirements
- LSTM models offer a good balance of performance and complexity
- BERT achieves the best results but requires significant computational resources
- Practical Considerations:
- For production systems, consider the trade-off between accuracy and inference time
- BERT’s superior performance might be worth the computational cost for accuracy-critical applications
- For real-time applications with limited resources, LSTM or even Word2Vec approaches might be more appropriate
- Future Directions:
- Explore domain-specific pre-training
- Investigate lightweight transformer architectures
- Consider multi-task learning approaches
The choice of model should ultimately depend on your specific use case, taking into account factors like accuracy requirements, computational resources, and latency constraints.
26 Feb 2020
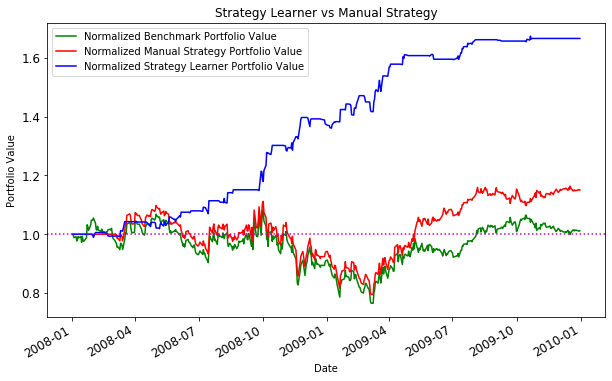
Project Link
1.Background
I spent the last 2 days to build an algorithm trading starter notebook. It is essentially using a different approach compared to what we are doing now for the FX trading project. I would like to have this as an alternative starting point and compare the performance between 2 different approaches. To make it easier for others to compare, I took the data scientist poor engineering practice to commit data into a repo. The main techniques are learned from the course Machine Learning for Trading, taught by Tucker Balch, who left Georgia Tech to work for JP Morgan now.
1.1 AWS setup
I would like to recommend my favourite setup, by using AWS Deeplearning AMI (Google Cloud or Azure are mostly the same based on my experience). A normal p2.xlarge would be more than sufficient. If you prefer to work with Jupyter notebook, Fast.ai has awesome documentation about the setup.
1.2 Alternative setup
Another highly recommended tool is Google’s colab. I almost always use it for an experiment. The only thing is we need a bit of setup to use Google drive to insert data. This post showed how to connect Colab to Gdrive.
2.Method
Financial data are normally time series data. So sequential models like LSTM is a naturally a good choice, just like we used in our internal project. But in this notebook, we embedded time-series information into technical indicators, then for each day, apart from price, there are several technical indicators taking historical information as part of the input. In this way, we can use frameworks like Gradient boosted trees or Q-learning to train our dataset.
2.1 Assumption
We assume the Efficient Market Hypothesis is not holding, or at least semi-strong or strong form do not hold. But it should be a common-sense for quantitative trading hedge fund like Renaissance Technologies. There should be some signals or correlations in stock prices, but not for all. We need some methods to find them out.
2.2 Pipeline Demo
The processing pipeline is shown in the README.md.
The target of the model is 3 positions: HOLD, BUY and SELL. Each day we have price information about one stock, with selected technical indicators containing historical information. We trained the model to understand the position to take for each day, then based on the positions, we can find the holdings. Subsequently, we use daily holdings to calculate the orders we should make. Eventually, our final product is a order book of days we BUY or SELL particular stocks.
2.2.1 Backtesting
The starting point of backtesting is orders file. We should treat backtesting separately, and it is probably the most important thing of the whole pipeline. What we need to make sure is that the backtesting result and forward testing result are similar. This is a crucial point. But not in the discussion of this post. This notebook is served as a starting point of exploration.
3.Result
The experiment results without too much fine-tuning are shared in the notebook.
In the experiment, the ML model is performed much better, but I set the risk free rate to 0 and market impact to the minimum. There many more concerns about the market environment. So to make sure the model would perform well in the real market, we need to spend extra effort in fine-tuning backtesting model.
4.Future work
There are several things I would like to try out to make this notebook starter more robust.
- Use deep reinforcement learning approach.
- Use more mature frameworks like LightGBM, and process with more data.
- Try stacking and other ensembling methods.
- Integrate with news data.
- Apply to Two Sigma’s kaggle competition
01 Jan 2020
Course Link
First of all, this is an easy course even for people like me with no prior Java experience. My final grade should be around 97%(A).
The course itself is great, containing many key concepts in software engineering. It even worth revisiting after the semester end. Syllabus shown here.
Also, I learned how to use IntelliJ and Android Studio for the semester projects. That’s a nice start since I always want to embeded tensorflow.js into an Android to build a ML based simple app. The most interesting experience I got from this course is to work distantly with 3 other teammates from the states. It’s definitely a culture shock. Basically the whole project is done asynchronously. Now I understand with the highly developed workflow, it’s totally possible to work remotely. Cutting off unnecessary meetings may not be a bad idea to boost productivity.
Finally, this might be a common problem for this master course. I need to consolidate information from all channels: Slack, Piazza, YouTube for relavant videos, and so on. The lecture itself is very interesting but the assignment will often take some extra efforts.
Finally, this is the recommended resources for this course:
- Git
- https://www.atlassian.com/git/tutorials
- https://github.com/progit/progit2
- Java
- https://www.codecademy.com/learn/learn-java
- https://www.guru99.com/java-tutorial.html
- IntelliJ
- https://www.youtube.com/watch?v=Bld3644bIAo
- https://www.youtube.com/watch?v=c0efB_CKOYo&list=PLPZy-hmwOdEXdOtXdFzyx_XCnrF_oD2Ft
- Android Studio
- https://www.youtube.com/watch?v=g9YblXBQ5uU&t=11s
- https://www.youtube.com/watch?v=dFlPARW5IX8&t=694s
- Flow Chart
06 Dec 2019
Course Link
Firstly, this course is very intense to include many topics in one semester. Especially, the weekly assignments are all hard problems, which would take majority of the study time. The ideal way is to go through all materials before the beginning of semester.
Eventually, I scored 89.27% for this course, almost a highest B one can get. I don’t really care about getting straight As, but this is a bit unfortunate since I was only 0.7% away.
The big lesson learnt is that, I should trust reviews and experiences. I’ve been warned multiple times from all channels that CNN project is a monster, but I still went with it since I thought I knew it well even before this course. But the reality is different. The final project is just so demanding and my past CNN experience is almost irrelevant. If I chose EAR or MHI, it would be definitely a much easier one.
Intro & Syllabus
Fortunately, I have a similar OCR project to do this semester, so some of the methods I do apply to my work. This could be the best case scenario for a part-time Master.